How can you run a class with big data?
- DeeDee Perrott
- Jan 28, 2021
- 4 min read
Updated: Feb 16, 2021
Strategies and concepts for how to use big data in the classroom.
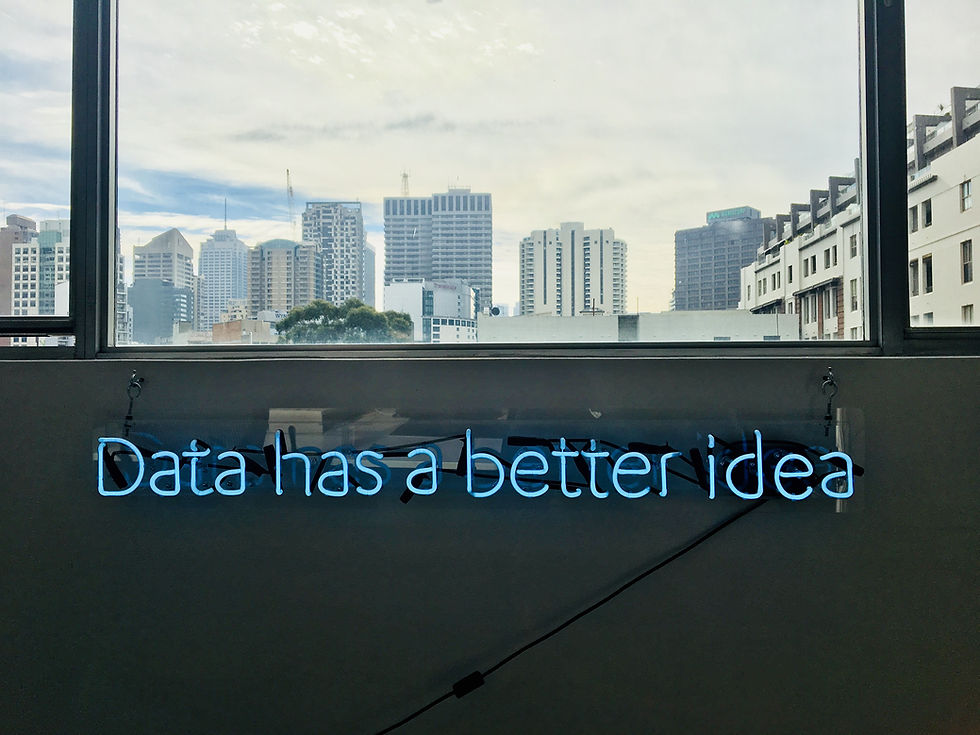
The educational world is a constantly shifting and dynamic place. With the advancement of technology has also come the advancement of education. Big data lives between both worlds, combining the powers of technology with the education of the next generation. Big data has the potential to revolutionize education, but the focus can not shift away from the individual needs and preferences of the student.
When we look at how to improve the learning of our students through the use of big data, there are three core qualities of big data that have this ability: feedback, individualizations, and probabilistic predictions (Mayer-Schönberger & Cukier, 2014).
Feedback
Traditionally, feedback has been a one-way street in education. This usually reflects how well a student has understood a lesson and is recorded and evaluated through some traditional form of assessment. This focus is then on the result of learning, rather than honing in on the learning process. This one-directional correspondence would be alike to manufacturers or retailers evaluating the customers, without any chance for the manufacturer or retailer to be evaluated themselves (Mayer-Schönberger & Cukier, 2014). If we open up the doors to allow feedback in both directions, not only will the student understand what they need to do differently or improve in order to be successful, but the teachers, administrators, and educational environments will also be evaluated and held accountable for the successes and failures of their systems. Big data in an educational setting can be a material used to help bridge this gap and form a multi-directional datified feedback relationship between students, teachers, administrators and educational environments.
Using big data collection and analysis in education parlays information to the students to improve comprehension and performance while also sharing this information and data with teachers, administrators, and other educational institutions to improve the educational systems. An example of this is through the use of e-books. With e-books, signals can be collected, processed, and used to give students feedback while also sending feedback to the teachers and the publishers. The student may use this feedback to extensify their learning and revisit material that they potentially have missed. The teacher may use this feedback to guide their next steps with lessons or to hone-in on materials that may have been missed by the students. And finally, the publisher may use this feedback to learn how students engaged with their material, what they enjoyed, and what they didn’t find useful (Mayer-Schönberger & Cukier, 2014). This process happens through something called clickstream data. Clickstream data has the potential to record where students are clicking, what they clicked in order to become successful, and what they didn’t click (Mayer-Schönberger & Cukier, 2014). This tracks the successful and unsuccessful paths of learning and provides the feedback to the students, teachers, and publishers with the intention of improving individual student learning. Eventually, with the help of adaptive learning, this analysis could happen in real time, automatically presenting students with materials that are the best fit for their specific needs in a particular moment. This leads to some highly personalized and individualized learning and instruction.
Individualization
The time of treating students alike, using identical materials and problems sets, and benchmarking learning against a set of standards that have been developed from averages is becoming a time of the past. These traditional educational methods lack individual preferences, qualities, and challenges (Mayer-Schönberger & Cukier, 2014). With the marriage of technology and education, the ability to individualize and tailor learning to each student’s needs and preferences is now more accessible. Shonberger and Cukier (2014) call this the “one size fits one” (p. 31) philosophy. Big data, paired with adaptive learning, allows educators, administrators, and educational institutions to fulfill this philosophy of learner first, individualized education.
An example of adaptive learning software that focuses on individualization is MATHia. MATHia is a high school mathematics software created by Carnegie Learning. This software aims to take mathematics beyond scores on standardized tests, and reinvent it as an area of learning focused on problem solving and deep conceptual understanding. MATHia uses adaptive learning that individualizes the learning experience by deciding which math questions to ask each student based on how each student answered the previous questions (Mayer-Schönberger & Cukier, 2014). It also has the ability to identify problem areas, and increase the practice in these areas versus continuing on to the next concept before the student is ready. Through the use of adaptive learning software such as MATHia, students learn at different paces, and at their own level. The learning materials are dynamic, adaptive, and transformative. The more data that is collected and analyzed, the more information that can be used as feedback to respond to the individual needs of the students (Mayer-Schönberger & Cukier, 2014).
Some other adaptive learning software that focuses on individualized learning experiences include:
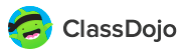
Probabilistic Predictions
Probabilistic predictions are an important facet of big data and education to take into account when considering its use in educational settings. Although it is possible to use big data to predict, with a high level of accuracy, what each individual student needs to do to improve their educational performance (materials, teaching, feedback mechanism), these are still only probabilistic predictions (Mayer-Schönberger & Cukier, 2014). Because of this, educational institutions and all involved need to recognize and appreciate the limitations. Big data and adaptive learning software do not offer certainty or guarantees. And this is important to consider given the high stakes of education. Decisions surrounding education, big or small, all have the ability to have a major effect on the future success of the students, the teachers, and the educational institutions (Mayer-Schönberger & Cukier, 2014).
With that said, big data functions in a world of quantification. The more data collected, the bigger the quantification, and this results in greater, more detailed precision. Big data requires the user to shift from a mindset of casualties (which education focuses on), to an ideology of correlations (Mayer-Schönberger & Cukier, 2014). Big data opens up the educational world to “seeming connections and associations among variables that we might not have otherwise known” (Mayer-Schönberger & Cukier, 2014, p. 40). As Shonberger and Cukier (2014) explain, “[these correlations] do not tell us why something is happening, only what is happening...but that is often good enough to help us make decisions” (p. 40).
That's a great question Meg! While the use of some adaptive learning software may be within reach for individual classes, harnessing big data in the individual classroom on a big scale seems unattainable without institutional support. This paper by UBC professor Leah MacFadyen (https://www.jstor.org/stable/10.2307/jeductechsoci.15.3.149) details some of their team's findings and challenges with the use of learning analytics to inform decision-making and guide strategic direction within an institution. It highlights the need to have the support of key stakeholders within an organization, including administrators and educators, in order to "motivate organizational adoption and cultural change."
These videos were great! My question is how do we as teachers access program that use big data in terms of cost, availability, alignment with provincial curriculum and that appease the privacy concerns of districts?